The first one is the Maximum Likelihood Estimator method (probabilistic approach). Knowing that p(w) does not vary, minimizing (-log p(y|X,w)p(w) ) is the same as minimizing (- log p(y|X,w)) which is maximizing the log-likelihood.
The third one is the dual representation of ridge regression, if you take the gradient with respect to w and set it to zero you will find that you can solve for w as a linear combination of all data points x. You can find the derivation in the lecture notes called “kernelized ridge regression”
Least squares
Hello,
Can you please provide some explanations about how these results were found ( the first and third ones)?
Thank you!
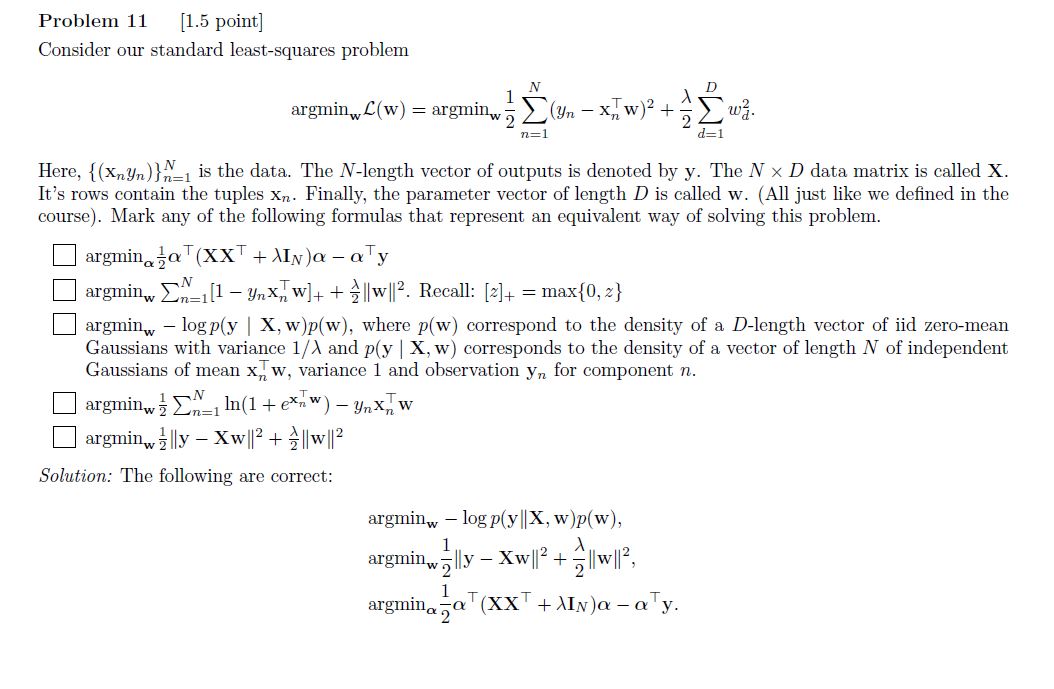
1
The first one is the Maximum Likelihood Estimator method (probabilistic approach). Knowing that p(w) does not vary, minimizing (-log p(y|X,w)p(w) ) is the same as minimizing (- log p(y|X,w)) which is maximizing the log-likelihood.
3
The third one is the dual representation of ridge regression, if you take the gradient with respect to w and set it to zero you will find that you can solve for w as a linear combination of all data points x. You can find the derivation in the lecture notes called “kernelized ridge regression”
2
Add comment